1. Introduction
Droughts are recurrent natural disasters characterized by a significant rainfall deficit, leading to water scarcity, streamflow depletion, crop damage, and groundwater reduction. They can be classified as meteorological, hydrological, agricultural, and socio-economic droughts (Dracup et al. 1980; Wilhite, Glantz 1985). Various parameters must be considered when defining the temporal and spatial scales and the regional characteristics of droughts (Tallaksen, Lanen 2004). More than 130 published drought definitions can be categorized in several ways, as noted by Wilhite and Glantz (1985). Some of the most commonly used definitions of droughts have been listed by Tate and Gustard (2000) and Demuth and Bakenhus (1994). Droughts have negative impacts on social life, the economy, and the environment, although these impacts are difficult to detect. Several indices have been developed to identify and analyze droughts, of which the most commonly used one is the standard precipitation index (SPI) (McKee et al. 1993; Ganguli, Reddy 2014). Other indices, such as the Palmer drought severity index (PDSI) (Palmer 1965), the surface water supply index (SWSI) (Shafer, Dezman 1982), and the crop moisture index (CMI) (Palmer 1968), are also widely applied to detect drought events.
Several drought trend studies have been conducted all over the world (Piccarreta et al. 2004; Xu et al. 2011), and the hydrological time series of precipitation, temperature, soil moisture, and evapotranspiration at the catchment scale have been widely investigated (Villarini et al. 2009; Burn et al. 2010; Gocic, Trajkovic 2013; Zhang et al. 2015). As a consequence of global warming and climate change, water resources, the environment, agricultural activities, and industrial production have been significantly affected (Shi, Xu 2008). However, the detection of changes in climate and hydrological time series is complex and challenging but has become an important issue because of the role of such changes in water resources management and drought analysis. According to the report of the Intergovernmental Panel on Climate Change (Houghton et al. 1996), in the 20th century, global land precipitation has increased by 2% on average. The impact of climate change on the maximum and minimum temperatures of Gombe City in northeastern Nigeria has shown an increasing trend (Alhaji et al. 2018). Asfaw et al. (2018) investigated trends in the precipitation and temperature parameters for the Woleka Basin in northern Ethiopia and found that the number of dry years increased. Although the mean and minimum temperatures presented an increasing trend, an insignificant trend was observed for the maximum temperature values. Keskin et al. (2015) studied monthly and annual water levels in Lake Eğirdir in Turkey, using Mann-Kendall and Sen’s slope tests. Based on their results, the annual water level was reduced by approximately 0.026 m. Karabulut et al. (2008) analyzed trends in rainfall and temperature data in the Samsun region between 1931 and 2006 and noted statistically significant trends in summer temperatures. Although there are numerous investigations on trends in hydrometeorological parameters and climate change (Cannarozzo et al. 2006; Yu et al. 2006; Kampata et al. 2008; Zhong, Li 2009; Kumar, Jain 2010; Santos et al. 2011; Yuce et al. 2015, 2018), only few studies have performed trend analyses in drought events (duration and severity) (Tabari et al. 2012; Ganguli, Reddy 2014; Spinoni et al. 2014; Dashtpagerdi et al. 2015; Zhang et al. 2015; Yuce et al. 2022).
As of the beginning of 2021, most of Turkey is facing a severe drought. An increase in the number of dry days is inevitable after several low-rainfall seasons. In 2019, the summer and autumn months were largely devoid of rain, resulting in decreased reservoir water levels. The year 2020 was the driest of the last 5 years, with particularly little rainfall in the latter half of the year, according to a NASA report1. This study will investigate the presence of any systematic changes in extreme drought events, using historical hydrometeorological data in the Ceyhan River Basin, which plays a critical role in the agricultural and hydropower production of the country. If the presence of any trend is detected, its magnitude and change point in the time series will be studied in detail. Furthermore, the trends of extreme drought events, such as the annual maximum drought severity (AMDS), defined as the largest cumulative severity value for each year, and the annual maximum drought duration (AMDD), which is described as the length of the maximum drought duration for each year, will be investigated. Notably, these two parameters are independent of one another. Here, the length of the AMDS may not be the same as the length of the AMDD within 1 year. Hence, AMDS and AMDD will be evaluated as different time series in statistical analysis tests. Both parameters of the extreme events are calculated by using the SPI method for multiple-time scales of 1, 3, 6, 9, and 12 months.
2. Study area and data
The Central Taurus Mountains are located in the northern and northwestern parts of the Ceyhan River Basin, with two different mountain ranges, namely the Bolkar Mountains and the Tahtalı Mountains starting from the west. The Ceyhan River Basin is situated in the Mediterranean Climate Zone, which is characterized by dry summers and mild, wet winters. The rainiest months are December, January, and February, and the driest months are June, July, and August. The total length of the Ceyhan River is around 425 km, with an annual discharge of 82.9 m3/s and a basin yield of 10.7 L/h/km3. The maximum total annual rainfall of the catchment is recorded in Kozan with at least 842 mm, and the lowest total annual rainfall is recorded in Elbistan with 395.7 mm (Eris et al. 2019; Yuce et al. 2019). The highest average annual temperature is 19.3°C in Kozan, whereas the lowest average annual temperature is 8.9°C in Göksun (Uzunkol, Kızılelma 2016).
For this study, we used long-term monthly precipitation data. To evaluate the SPI index, the precipitation data of 11 out of 23 meteorological stations were obtained from the General Directorate of Meteorology of Turkey (MGM), whereas the data of the other 12 stations were obtained from the General Directorate of the State Hydraulic Works of Turkey (DSI). The locations of 23 meteorological stations are displayed in Figure 1. The selected monthly precipitation time series with recorded lengths ranging from 15 years (station 7767) to 54 years (station 17255) for the period from 1963-2016 were employed in the analysis. This is a significant amount of data for extreme drought investigation in this basin. The statistical characteristics determined from the monthly precipitation time series for each gauging station are presented in Table 1. The mean, standard deviation, coefficient of variation (Cv), coefficient of skewness (Cs), and lag-one autocorrelation coefficient (r1) were evaluated by using the observed monthly precipitation time series.
Table 1
Statistical parameters of observed rainfall stations; S.D., standard deviation; Cv, coefficient of variance; Cs, skewness; r1, kurtosis.
3. Methodology
3.1. Standardized Precipitation Index
The SPI method, developed by McKee et al. (1993), is used to evaluate the level of deficiency in precipitation on different time scales ranging from 1 to 48 months. It is the most well-known and used index among many other indices to identify meteorological drought events and is based on fitting precipitation data to a probability distribution function (PDF). Gamma is the most suitable probability density distribution function for climatological data (Ganguli, Reddy 2014; Zhang et al. 2015). The dry period is considered to be the period in which the index is less than or equal to –1. The SPI, which is classified based on values presented in Table 2, is determined by Equation 1:
where Xij is the monthly precipitation amount and Xim and σ are the mean and standard deviation of precipitation evaluated from the all-monthly time series, respectively.
Table 2
SPI classification (McKee et al. 1993); SPI = Standardized Precipitation Index.
SPI value | Category |
---|---|
≥ 2 | Extremely wet |
1.50 to 1.99 | Very wet |
1.0 to 1.49 | Moderately wet |
0.99 to 0 | Normal |
0 to 0.99 | Near normal |
–1.00 to –1.49 | Moderately dry |
–1.50 to –1.99 | Severely dry |
≤ –2 | Extremely dry |
3.2. Homogeneity test
3.2.1. Wallis-Moore and Wald-Wolfowitz
The Wallis and Moore phase frequency test (Wallis, Moore 1941) is used to detect deviations of time series for randomness in the sequence of values. The test is based on sign differences (- or +), while the first and last phases named sequence of signs are not accounted thus the number of phases is identified. If n ≥10 and continuity correction is applied, a fairly good test may be based on the hypothesis that the data are normally distributed, when n ≥25, the correction is not used (Wallis, Moore 1941). The z test statistic is calculated as follows:
where h is the number of phases, although the first and last phases are not taken into account. The z-statistic is normally distributed. A continuity correction of –0.5 is added to the denominator for n ≤ 30.
The Wald-Wolfowitz test, which is also known as Runs test, measures the randomness of the data and examines whether an observation influences the subsequent observation. Time series data are cut from a certain level that could be the mean, medium, or mode to determine if each value in the series is lower or higher than this level. The number of passes from one data to the other above or below a certain level is called the run number, it is small if it is below or above long periods. Such series may not have homogeneity (Wald, Wolfowitz 1940). The result of the test is z, the number of data N, the number of runs r, the number of values below the medium level Na, the number of values above the medium level Nu; the equations are as follows:
3.3. Trend Analysis
3.3.1. Mann-Kendall Test
The Mann-Kendall test is a non-parametric test to determine the trend in the hydrometeorological variable in a time series (Mann 1945; Kendall 1975; Helsel et al. 2002). It is the most preferred statistical method for time series since it is not fitted to any distribution. The application of the Mann-Kendall test statistic Z is expressed as follows:
where n is the number of the data, xj and xk are the data point in years j and k (j > k), and ti is the length of the tied rank group.
A positive Z value indicates an increasing trend, whereas a negative value indicates a decreasing trend. Critical test statistical values are 1.645, 1.97, and 2.57 for different significance levels of 90%, 95%, and 99%, respectively (Yu et al. 1993).
3.3.2. Spearman’s Rho Method
Spearman’s rho test is a commonly employed nonparametric method to investigate the presence of trends (Lehmann, D’Abrera 1975; Sneyers 1990). Its statistics rs and z (standardized test statistics) are computed as follows:
where Rxi (rank statistic) is obtained by sorting the data, and n is the length of the time series. Negative z values show decreasing trends, whereas positive z values indicate increasing trends. At the 90% significance level, for z > ±1.645, the null hypothesis of no trend is rejected.
3.4. Trend Slope
3.4.1. Linear Regression Method
Regression analysis is a statistical method used to predict the relationships between one dependent variable and one or more independent variables. It forms the basis of complex estimation methods and is used to evaluate the strength of the relationship between variables and to model the relationship between them. The dependent variable is usually represented by Y, and the independent variables are denoted by X (Gocic, Trajkovic 2013). The linear regression formula is given as follows:
where a is the intercept, and b is the slope of the line. A positive b value indicates increasing trends, and a negative a value indicates decreasing trends.
3.4.2. Sen’s Slope Estimator
Sen’s slope test (Sen 1968) is a non-parametric test that calculates the slope of the trend in a data set; it is used for equiponderant time series. For each data point, the slope difference is calculated per changing time. The slope of the trend can be estimated by the median of all slopes between data pairs in the same season (Helsel et al. 2002). All slope pairs are ranked from smallest to largest, and if the calculated number of slopes (n) is odd, the median slope gives the slope S. If n is even, the two median slopes are averaged. Here, Q represents data, n is the length of the data, and T is time. The slope of n pair of data is predicted as follows:
3.5. Tests for Change Point Detection
Considering the importance of climatic variability in terms of water availability, increasing irrigation demand, crop yields, and other factors, it is crucial to identify and evaluate the trends in the monthly, seasonal, and annual historical data series. Change point detection is substantial to evaluate the period in which a significant change occurs in a time series. In this study, the standard normal homogeneity test (SNHT), Buishand’s range test (BRT), and Pettitt’s test (PT) were employed to detect change points in the time series.
3.5.1. Standard Normal Homogeneity Test
The statistic of the SNHT (Alexandersson 1986), (Tk), is used to compare the average of the first n year with the average of the last (n – k) year with n data points (Vezzoli et al., 2012; Jaiswal et al., 2015). The Tk equation is as follows:
Z1 and Z2 can be calculated as follows:
Here,
Table 3
Critical values for different change point detection test statistics.
3.5.2. Buishand´s Range Test
The adjusted partial sum, Sk, is computed as follows:
When the series is homogeneous, the value of Sk fluctuates around zero. The test captures data showing sensitivity to break in the middle of distorting the homogeneity of the time series. If there is a change in K year, it reaches the maximum or minimum value around the year k = K (Buishand 1982). The significant change can be computed by evaluating the rescale-adjusted range R:
3.5.3. Pettitt’s Test
PT is a non-parametric method (Pettitt 1979) to determine change detection in a time series. It is commonly used to calculate the occurrence of an unexpected change in hydrometeorological records (Sneyers 1990; Smadi, Zghoul 2006). The null hypothesis indicates that the series has an independent and random distribution, whereas the alternative hypothesis indicates a sudden change. The Uk test statistic is expressed as follows:
The Uk test results are presented graphically. In case of a break in k = K year, the test statistic is at maximum or minimum values. Significant critical values are given in Table 3.
4. Analysis of Results
4.1. Homogeneity Analyses
The precipitation data of 23 meteorological stations in the Ceyhan River Basin were analyzed by the SPI drought index for time scales of 1, 3, 6, 9, and 12 months. The AMDS and AMDD time series for station 17355 are illustrated in Figure 2. These graphs do not show any statistically significant trend in the data and provide only a rough view of the variation of the data over time.
The Wallis and Moore phase-frequency test and the Wald-Wolfowitz test were employed for the analysis of extreme drought events (AMDS and AMDD), verifying the homogeneity for different time scales. The results of these two tests were compared to achieve an accurate homogeneity and randomness in the time series. If the outcomes of both tests suggest homogeneity in the time series, the presence of homogeneity is accepted. When only one of the methods suggests homogeneity of the time series, the results are defined as doubtful; if both methods suggest no homogeneity in the data set, it is labeled as non-homogeneous. The homogeneity test results for the AMDS and the AMDD time series of 23 meteorological stations for time scales of 1, 3, 6, 9, and 12-month are presented in Table 4.
In the AMDS time series, for SPI 1-month time scale, the data of 19 out of 23 stations indicated homogeneity by both tests, whereas stations 17871, 17979, and D20A011 presented homogeneity by one of the methods. Only station 17908 showed non-homogeneity by both tests for the significance level of 90%. For the same time scale, stations 8275, 17355, 17870, 17908, and D20A016 presented homogeneity by one of the methods, and only station D20A006 illustrated non-homogeneity by both methods; the data for the remaining stations were homogeneous in the AMDD time series. The AMDS time series were homogenous in all stations for the SPI 3-month time scale, apart from station 17960, which presented homogeneity by the Wald-Wolfowitz test. Although the AMDS time series presented homogeneity by one of the methods at 10 stations (17866, 17870, 17960, 17979, D20A002, D20A009, D20A011, D20A013, D20A015, and D20A016), the data at only one station (8275) showed non-homogeneous characteristics. The data at the other stations showed homogeneous features.
Fig. 2.
The extreme drought events´ both AMDS and AMDD time series of station 17355 at different time scales.
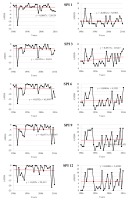
The SPI 6-month time scale AMDS time series presented homogeneity by one of the methods at stations 8275, 17868, and D20A002, whereas the other stations indicated homogenous data sets. The AMDD time series displayed non-homogenous features at stations 8275 and D20A013, homogeneous features by one of the methods at stations 17868, D20A016, and D20A017, and the data sets at the other stations were homogeneous by the two tests. With the increase in the length of the time scales, the number of non-homogenous stations also increased. For instance, three and five stations were non-homogenous for the SPI 9-month time scale and the SPI 12-month time scale, respectively. Station 17868 in both AMDS and AMDD as well as stations D20A001 and D20A016 in the AMDD time series were non-homogenous for the SPI 9-month time scale. Station 17868 in AMDS, stations D20A011 and D20A013 in both AMDS and AMDD, and stations D20A009 and D20A016 in the AMDD time series were non-homogenous for the SPI 12-month time scale.
Table 4
Homogeneity test results for extreme drought events at different time scales.
H: Homogeneous, D: Doubtful, N-H: Non-homogeneous
4.2. Trend Analyses
Trend detection analysis of extreme drought event time series under the influence of climate change is critical to propose and make use of management strategies. Table 5 summarizes the outcomes of the Spearman Rho and Mann-Kendall statistical tests used to detect any trends in the SPI 1-,3-,6-,9-, and 12-month time series. The null hypothesis is not rejected at the 90% significance level where –1.64 < z < 1.64. The Mann-Kendall and Spearman Rho methods performed similarly in the analysis of trend detection in the extreme drought event time series (Table 5). For the SPI 1-month time scale, a significant trend was not detected by both tests in the extreme drought events, except in the data of stations 17868 and D20A014. Although two tests showed a statistically significant increasing trend in the AMDS series of station17868, the AMDD series of station D20A014 showed a statistically significant decreasing trend. In the SPI 3-month time scale, the statistically significant increasing trend was evaluated only in the AMDS series of station 7767 and in the AMDD series of station 8275. In the case of the SPI 6-month time scale, the time series at four stations showed statistically significant trends in both test results. The AMDS and AMDD time series at station 7767 showed increasing and decreasing trends, respectively. Statistically significant increasing trends were observed in the AMDS time series of stations 8275, 17866, and D20A016, whereas decreasing trends were noted in the AMDS time series of station D20A016, with a significance level of 90%. As in the SPI 3-month period, similar results were clearly seen for the SPI 9-month time scale of stations 7767, 8275, and D20A016. In the time series at five stations, statistically significant trends were determined for the SPI 12-month time scale. Increasing trends were perceived in the AMDS time series of stations 7767 and D20A018, whereas decreasing trends were detected in the AMDS time series of stations 8275 and D20A016. Furthermore, both tests demonstrated a statistically significant increasing trend in the AMDD time series of stations 8275 and D20A009.
Table 5
Trend detection results for extreme drought events.
N: No Trend, D: Decreasing, I: Increasing, MK: Mann-Kendall, SR: Spearman Rho
4.3. Trend Magnitude
Sen’s slope estimator and linear regression analysis were employed to determine the slope of trends in the AMDS and AMDD time series. The signs of the slopes were in line with the results of the Spearman Rho and Mann-Kendall tests. The slopes of the trend lines are indicated in bold in the time series where the trend was detected (Table 6). The highest slopes of the trend line, ranging from 0.6 to 0.8 for both tests, were detected in the AMDS time series of station 7767 for the SPI 9-month time scale. The other evaluated slopes of the trend were 0.067 in AMDD time series of station 8275 for the SPI 3-month time scale, 0.179 in the AMDD time series of station 8275 for the SPI 6-month period, 0.106 in the AMDD time series of station 8275 for the SPI 9-month period, 0.083 in AMDS time series of station 8275 for the SPI 12-month period, and 0.138 in the AMDD time series of station 8275 for the SPI 12-month period.
Table 6
Trend Magnitude Test results for all stations.
4.4. Change Point Detection
After determining the presence of a trend as well as its slope in the time series of extreme drought events, SNHT, BRT, and PT were applied to define the point where the change had started. The change point is identified as the point where the change point occurs in SNHT and BRT, which can be depicted graphically. The outcomes of the three methods were compared to assess a precise change point. As two of the three tests presented the same results, that specific point was chosen to be the change point for the particular time series. However, if the outcomes of all three tests were inconsistent, the change point selection was denoted as suspicious (S). The change points for extreme drought events of the AMDS and AMDD time series are presented in Table 7.
Table 7
Change point results of extreme drought events.
NC: No change
All three tests were performed with a significance level of 90%. According to test results, no abrupt changes were found at station 7767 for the SPI 1-month period in the AMDS and AMDD time series, the SPI 3-month period in the AMDD time series, and the SPI 12-month period in the AMDD time series. Changes in the AMDS time series were noted in the year 2000 for SPI 3-, in 2001 for SPI 6-, 9-, and 12-month time scales. Changes in the AMDD series were found in 2008 for SPI 6- and SPI 9- at station 7767. We detected no changes in both the AMDS and AMDD time series at station D20A014. Changes were observed in 1981 at station 17866 for the SPI 6- in the AMDD time series, in 1982 at station D20A009 for the SPI 12- in the AMDD time series, and in 1988 at station D20A018 for the SPI 12-AMDD time series (Fig. 3).
In general, numerous change points were detected at stations 7767, 8275, and D20A016, with a significance level of 90%. Although there were no changes for the SPI 1-month time scales and the SPI 3-month time scales at station 8275, the years 1979, 1969, and 1980 were critical for the SPI 6-AMDS time series, the SPI 9-AMDD time series, and the SPI 12-AMDS time series, respectively. At station D20A016, the change point was detected in 1988, which was a critical year for the SPI 6-AMDS and AMDD time series, for the SPI 9-AMDS and AMDD time series, and for the SPI 12-AMDS time series.
5. Discussion and Conclusions
The AMDS and the AMDD trends in extreme drought events were evaluated in this study. The SPI drought index was used to assess the extreme events for 23 meteorological stations in Ceyhan Basin over several time scales of 1, 3, 6, 9, and 12 months. The AMDS and AMDD time series are important extreme hydrological drought events in disaster preparedness and the regional management of water supplies. The homogeneity of the extreme drought occurrence time series was investigated using the Wallis-Moore and Wald-Wolfowitz methods, and Mann-Kendal and Spearman Rho trend analyses were carried out. Sen’s slope estimator and linear regression analysis were used to assess the magnitude of trends, and the SNHT, BRT, and PT were used to identify the change point that denotes the start of the trend.
The number of stations with non-homogeneous data increased as the SPI time scales increased, especially in the AMDS time series. Most of the stations seemed to have homogeneous time series; however, the data in a few stations were homogeneous by one of the methods and non-homogeneous by the other one, with a significance level of 90%.
In the AMDS series, an increasing trend was observed only in the station 7767 data for 3-, 6-, 9-, and 12-month time scales, whereas decreasing trends were detected in station 8275 for the 12-month time scale and in station D20A016 for the 6-, 9-, and 12-month time scales. Nevertheless, in the AMDD time series, increasing trends were noted in station 8275 for the 3-, 6-, 9-,and 12-month time scales, station 17866 for the 6-month time scale, station D20A009 for the 12-month time scale, station D20A016 for the 6- and 9-month time scales, and station D20A018 for the 12-month time scale, whereas decreasing trends were perceived in the station 7767 for the 6- and 9-month time scales and in station D20A014 for the 1-month time scale.
These results indicate that the AMDD time series with higher time scales revealed an increasing trend, whereas the AMDS time series showed a decreasing trend, which was statistically significant at the 90% confidence level.
Possible change points started after 1988 for D20A016 and D20A018, whereas the data in stations 7767, 8275, 17866, and D20A009 shifted after late 2000, 1969, 1981, and 1982, respectively. Change point detection tests captured different years in the data of some stations, most likely because of climate type, topography, and hydrological conditions, among others. Investigations on drought and climate change at local scales need to be periodically repeated to assess the impacts of precipitation, temperature, evapotranspiration, and groundwater level on agriculture, water resources and food security, energy production and security, economic growth, ecosystem sustainability, environmental health, and health security. The results of this study are consistent with previous findings. For instance, Di̇ki̇ci̇ and Aksel, (2021) indicated that precipitation is generally decreasing, considering a 50-year period. According to their calculations, the basin faces a severe drought threat in the 20-year return period. Tosunoglu and Kisi (2016) investigated the trends of maximum hydrologic drought variables, annual maximum duration (AMD), and annual maximum severity (AMS) using MK, modified MK, and the recently developed Sen’s innovative trend analysis (ITA) techniques. Increasing trends in the severity of the drought at the stations 2316 and 2323 were captured by the modified MK and ITA techniques, respectively. The possibility of future droughts or water shortages in these stations should be considered. The results of this paper provide valuable information to water resource management decision-makers in the Ceyhan River Basin for evaluating the effects of droughts and preparing for drought mitigation measures to avoid future drought risks.